Artificial Intelligence (AI) and machine vision technologies are rapidly transforming industries by automating complex visual inspections and quality control processes. However, despite their potential, many AI vision projects fail to deliver the expected results. Understanding the common pitfalls and how to avoid them can significantly increase the chances of success for your AI vision initiatives.
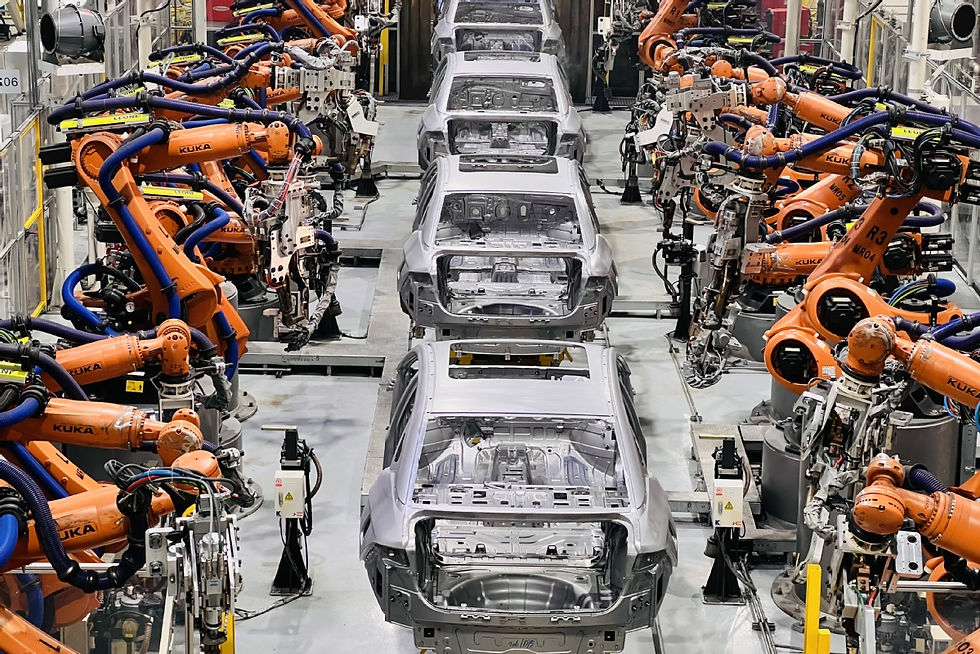
Common Reasons for Failure
Inadequate Data Quality and Quantity
AI models rely heavily on the quality and quantity of training data. Insufficient or poor-quality data can lead to inaccurate models that fail to generalize well in real-world scenarios. For vision systems, this often means not having enough labeled images representing all possible variations and defects that the system will encounter in production.
Unrealistic Expectations
Many projects fail because stakeholders have unrealistic expectations of what AI vision systems can achieve. AI is not a magic solution; it requires proper setup, training, and continuous improvement. Overpromising on capabilities or underestimating the time and resources required can lead to disappointment and project abandonment.
Lack of Domain Expertise
AI vision projects often require a combination of domain expertise and technical knowledge. Without the right expertise, it's challenging to define the appropriate inspection criteria, select the right AI models, and properly label training data. This can result in a system that doesn't meet the specific needs of the application.
Integration Challenges
AI vision systems need to be integrated into existing production lines and workflows. Integration challenges, such as compatibility with existing hardware and software, or the ability to communicate with other systems, can cause delays and complications that derail a project.
Neglecting Continuous Improvement
AI models need to be continuously updated and retrained as new data becomes available. Neglecting this aspect can lead to model degradation over time, where the system becomes less effective at detecting defects or performing inspections accurately.
How to Ensure Success
Invest in High-Quality Data
Ensure you have a diverse and comprehensive dataset that covers all possible variations and defects your system will encounter. Spend time on proper labeling and consider augmenting your dataset to improve model robustness. The more representative your data, the better your AI model will perform.
Set Realistic Goals
Clearly define what you want to achieve with your AI vision project and set realistic goals based on your current capabilities. Understand that AI will require iteration and improvement over time, and set expectations accordingly with all stakeholders.
Leverage Domain Expertise
Involve subject matter experts in your project from the start. Their insights will be crucial in defining inspection criteria, labeling data correctly, and choosing the right AI models. Collaboration between AI experts and domain specialists is key to creating a system that truly meets the needs of your application.
Plan for Integration
Consider the integration requirements from the outset. Ensure that your AI vision system can be seamlessly incorporated into your existing production environment. This might involve choosing hardware that is compatible with your current setup or developing custom software to bridge any gaps.
Focus on Continuous Improvement
AI is not a set-it-and-forget-it technology. Plan for continuous model updates and improvements. Regularly retrain your models with new data to ensure they remain accurate and effective. Establish a feedback loop where operators can flag missed defects or false positives to further refine the system.
Conclusion
AI vision projects have the potential to revolutionize manufacturing and quality control, but they come with their own set of challenges. By understanding the common reasons for failure and taking proactive steps to address them, you can increase the likelihood of a successful implementation. Focus on high-quality data, realistic expectations, domain expertise, seamless integration, and continuous improvement to ensure your AI vision system delivers the results you need.
Komentáře